Note
Go to the end to download the full example code. or to run this example in your browser via Binder
Digits Classification Exercise#
A tutorial exercise regarding the use of classification techniques on the Digits dataset.
This exercise is used in the clf_tut part of the supervised_learning_tut section of the stat_learn_tut_index.
KNN score: 0.961111
LogisticRegression score: 0.933333
# Authors: The scikit-learn developers
# SPDX-License-Identifier: BSD-3-Clause
from sklearn import datasets, linear_model, neighbors
X_digits, y_digits = datasets.load_digits(return_X_y=True)
X_digits = X_digits / X_digits.max()
n_samples = len(X_digits)
X_train = X_digits[: int(0.9 * n_samples)]
y_train = y_digits[: int(0.9 * n_samples)]
X_test = X_digits[int(0.9 * n_samples) :]
y_test = y_digits[int(0.9 * n_samples) :]
knn = neighbors.KNeighborsClassifier()
logistic = linear_model.LogisticRegression(max_iter=1000)
print("KNN score: %f" % knn.fit(X_train, y_train).score(X_test, y_test))
print(
"LogisticRegression score: %f"
% logistic.fit(X_train, y_train).score(X_test, y_test)
)
Total running time of the script: (0 minutes 0.055 seconds)
Related examples
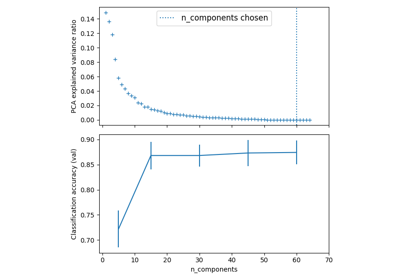
Pipelining: chaining a PCA and a logistic regression
Pipelining: chaining a PCA and a logistic regression
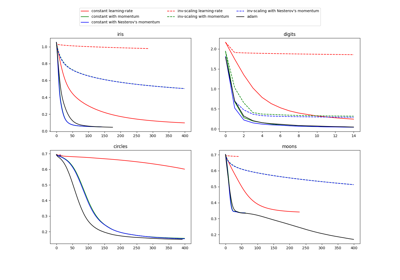
Compare Stochastic learning strategies for MLPClassifier
Compare Stochastic learning strategies for MLPClassifier
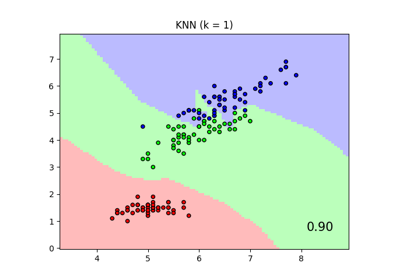
Comparing Nearest Neighbors with and without Neighborhood Components Analysis
Comparing Nearest Neighbors with and without Neighborhood Components Analysis