load_digits#
- sklearn.datasets.load_digits(*, n_class=10, return_X_y=False, as_frame=False)[source]#
Load and return the digits dataset (classification).
Each datapoint is a 8x8 image of a digit.
Classes
10
Samples per class
~180
Samples total
1797
Dimensionality
64
Features
integers 0-16
This is a copy of the test set of the UCI ML hand-written digits datasets https://archive.ics.uci.edu/ml/datasets/Optical+Recognition+of+Handwritten+Digits
Read more in the User Guide.
- Parameters:
- n_classint, default=10
The number of classes to return. Between 0 and 10.
- return_X_ybool, default=False
If True, returns
(data, target)
instead of a Bunch object. See below for more information about thedata
andtarget
object.Added in version 0.18.
- as_framebool, default=False
If True, the data is a pandas DataFrame including columns with appropriate dtypes (numeric). The target is a pandas DataFrame or Series depending on the number of target columns. If
return_X_y
is True, then (data
,target
) will be pandas DataFrames or Series as described below.Added in version 0.23.
- Returns:
- data
Bunch
Dictionary-like object, with the following attributes.
- data{ndarray, dataframe} of shape (1797, 64)
The flattened data matrix. If
as_frame=True
,data
will be a pandas DataFrame.- target: {ndarray, Series} of shape (1797,)
The classification target. If
as_frame=True
,target
will be a pandas Series.- feature_names: list
The names of the dataset columns.
- target_names: list
The names of target classes.
Added in version 0.20.
- frame: DataFrame of shape (1797, 65)
Only present when
as_frame=True
. DataFrame withdata
andtarget
.Added in version 0.23.
- images: {ndarray} of shape (1797, 8, 8)
The raw image data.
- DESCR: str
The full description of the dataset.
- (data, target)tuple if
return_X_y
is True A tuple of two ndarrays by default. The first contains a 2D ndarray of shape (1797, 64) with each row representing one sample and each column representing the features. The second ndarray of shape (1797) contains the target samples. If
as_frame=True
, both arrays are pandas objects, i.e.X
a dataframe andy
a series.Added in version 0.18.
- data
Examples
To load the data and visualize the images:
>>> from sklearn.datasets import load_digits >>> digits = load_digits() >>> print(digits.data.shape) (1797, 64) >>> import matplotlib.pyplot as plt >>> plt.matshow(digits.images[0], cmap="gray") <...> >>> plt.show()
Gallery examples#
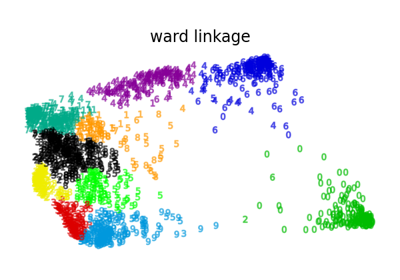
Various Agglomerative Clustering on a 2D embedding of digits
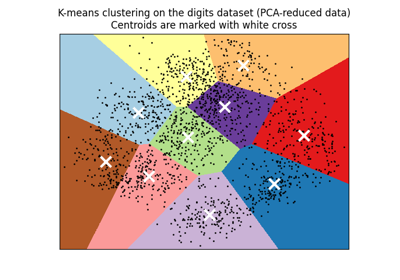
A demo of K-Means clustering on the handwritten digits data
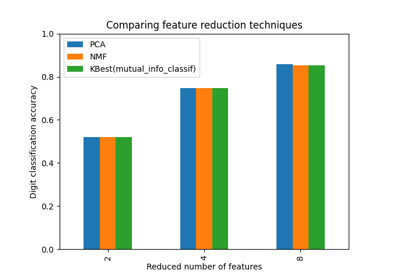
Selecting dimensionality reduction with Pipeline and GridSearchCV
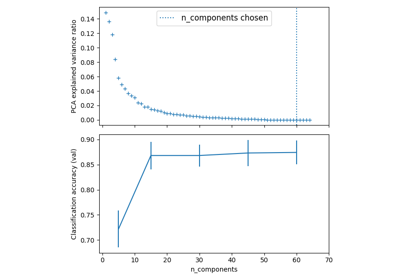
Pipelining: chaining a PCA and a logistic regression
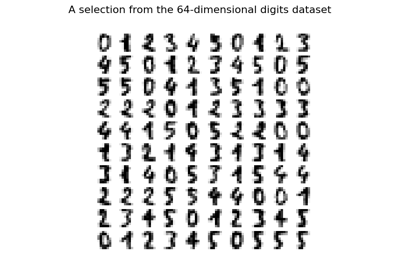
Manifold learning on handwritten digits: Locally Linear Embedding, Isomap…
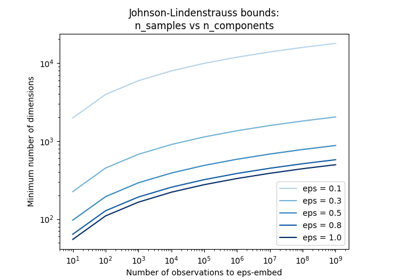
The Johnson-Lindenstrauss bound for embedding with random projections

Explicit feature map approximation for RBF kernels
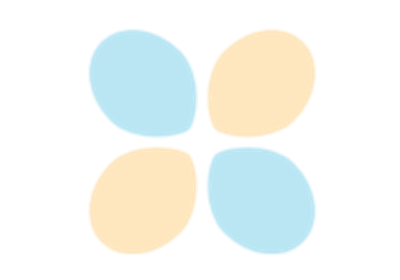
Custom refit strategy of a grid search with cross-validation

Balance model complexity and cross-validated score
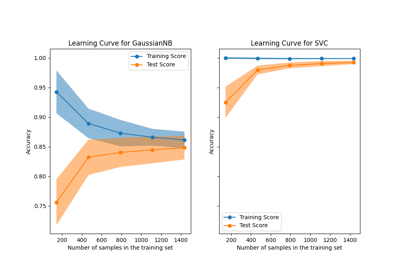
Plotting Learning Curves and Checking Models’ Scalability
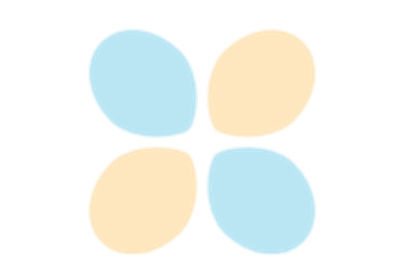
Comparing randomized search and grid search for hyperparameter estimation
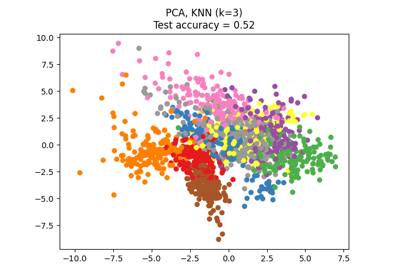
Dimensionality Reduction with Neighborhood Components Analysis
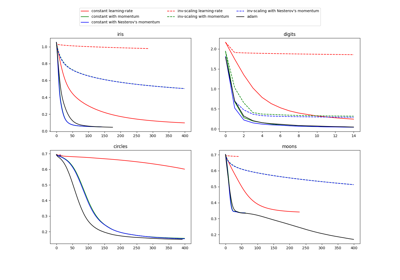
Compare Stochastic learning strategies for MLPClassifier

Restricted Boltzmann Machine features for digit classification
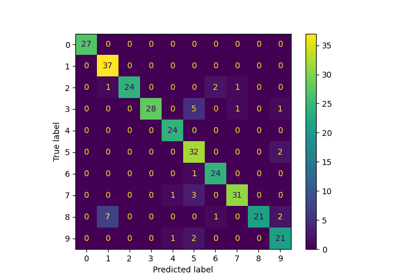
Label Propagation digits: Demonstrating performance