Note
Go to the end to download the full example code. or to run this example in your browser via Binder
Label Propagation digits active learning#
Demonstrates an active learning technique to learn handwritten digits using label propagation.
We start by training a label propagation model with only 10 labeled points,
then we select the top five most uncertain points to label. Next, we train
with 15 labeled points (original 10 + 5 new ones). We repeat this process
four times to have a model trained with 30 labeled examples. Note you can
increase this to label more than 30 by changing max_iterations
. Labeling
more than 30 can be useful to get a sense for the speed of convergence of
this active learning technique.
A plot will appear showing the top 5 most uncertain digits for each iteration of training. These may or may not contain mistakes, but we will train the next model with their true labels.
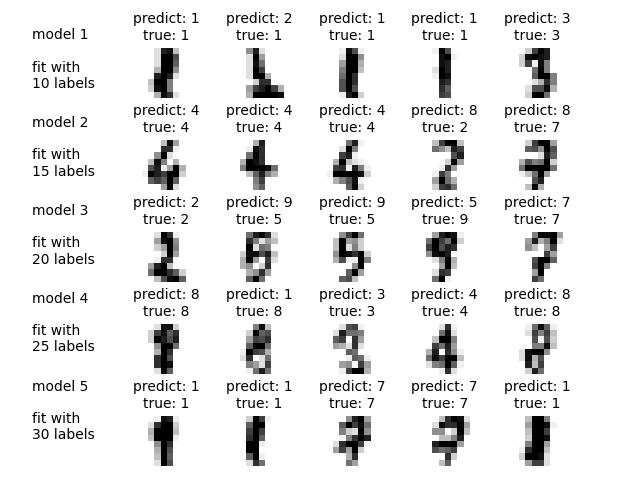
Iteration 0 ______________________________________________________________________
Label Spreading model: 40 labeled & 290 unlabeled (330 total)
precision recall f1-score support
0 1.00 1.00 1.00 22
1 0.78 0.69 0.73 26
2 0.93 0.93 0.93 29
3 1.00 0.89 0.94 27
4 0.92 0.96 0.94 23
5 0.96 0.70 0.81 33
6 0.97 0.97 0.97 35
7 0.94 0.91 0.92 33
8 0.62 0.89 0.74 28
9 0.73 0.79 0.76 34
accuracy 0.87 290
macro avg 0.89 0.87 0.87 290
weighted avg 0.88 0.87 0.87 290
Confusion matrix
[[22 0 0 0 0 0 0 0 0 0]
[ 0 18 2 0 0 0 1 0 5 0]
[ 0 0 27 0 0 0 0 0 2 0]
[ 0 0 0 24 0 0 0 0 3 0]
[ 0 1 0 0 22 0 0 0 0 0]
[ 0 0 0 0 0 23 0 0 0 10]
[ 0 1 0 0 0 0 34 0 0 0]
[ 0 0 0 0 0 0 0 30 3 0]
[ 0 3 0 0 0 0 0 0 25 0]
[ 0 0 0 0 2 1 0 2 2 27]]
Iteration 1 ______________________________________________________________________
Label Spreading model: 45 labeled & 285 unlabeled (330 total)
precision recall f1-score support
0 1.00 1.00 1.00 22
1 0.79 1.00 0.88 22
2 1.00 0.93 0.96 29
3 1.00 1.00 1.00 26
4 0.92 0.96 0.94 23
5 0.96 0.70 0.81 33
6 1.00 0.97 0.99 35
7 0.94 0.91 0.92 33
8 0.77 0.86 0.81 28
9 0.73 0.79 0.76 34
accuracy 0.90 285
macro avg 0.91 0.91 0.91 285
weighted avg 0.91 0.90 0.90 285
Confusion matrix
[[22 0 0 0 0 0 0 0 0 0]
[ 0 22 0 0 0 0 0 0 0 0]
[ 0 0 27 0 0 0 0 0 2 0]
[ 0 0 0 26 0 0 0 0 0 0]
[ 0 1 0 0 22 0 0 0 0 0]
[ 0 0 0 0 0 23 0 0 0 10]
[ 0 1 0 0 0 0 34 0 0 0]
[ 0 0 0 0 0 0 0 30 3 0]
[ 0 4 0 0 0 0 0 0 24 0]
[ 0 0 0 0 2 1 0 2 2 27]]
Iteration 2 ______________________________________________________________________
Label Spreading model: 50 labeled & 280 unlabeled (330 total)
precision recall f1-score support
0 1.00 1.00 1.00 22
1 0.85 1.00 0.92 22
2 1.00 1.00 1.00 28
3 1.00 1.00 1.00 26
4 0.87 1.00 0.93 20
5 0.96 0.70 0.81 33
6 1.00 0.97 0.99 35
7 0.94 1.00 0.97 32
8 0.92 0.86 0.89 28
9 0.73 0.79 0.76 34
accuracy 0.92 280
macro avg 0.93 0.93 0.93 280
weighted avg 0.93 0.92 0.92 280
Confusion matrix
[[22 0 0 0 0 0 0 0 0 0]
[ 0 22 0 0 0 0 0 0 0 0]
[ 0 0 28 0 0 0 0 0 0 0]
[ 0 0 0 26 0 0 0 0 0 0]
[ 0 0 0 0 20 0 0 0 0 0]
[ 0 0 0 0 0 23 0 0 0 10]
[ 0 1 0 0 0 0 34 0 0 0]
[ 0 0 0 0 0 0 0 32 0 0]
[ 0 3 0 0 1 0 0 0 24 0]
[ 0 0 0 0 2 1 0 2 2 27]]
Iteration 3 ______________________________________________________________________
Label Spreading model: 55 labeled & 275 unlabeled (330 total)
precision recall f1-score support
0 1.00 1.00 1.00 22
1 0.85 1.00 0.92 22
2 1.00 1.00 1.00 27
3 1.00 1.00 1.00 26
4 0.87 1.00 0.93 20
5 0.96 0.87 0.92 31
6 1.00 0.97 0.99 35
7 1.00 1.00 1.00 31
8 0.92 0.86 0.89 28
9 0.88 0.85 0.86 33
accuracy 0.95 275
macro avg 0.95 0.95 0.95 275
weighted avg 0.95 0.95 0.95 275
Confusion matrix
[[22 0 0 0 0 0 0 0 0 0]
[ 0 22 0 0 0 0 0 0 0 0]
[ 0 0 27 0 0 0 0 0 0 0]
[ 0 0 0 26 0 0 0 0 0 0]
[ 0 0 0 0 20 0 0 0 0 0]
[ 0 0 0 0 0 27 0 0 0 4]
[ 0 1 0 0 0 0 34 0 0 0]
[ 0 0 0 0 0 0 0 31 0 0]
[ 0 3 0 0 1 0 0 0 24 0]
[ 0 0 0 0 2 1 0 0 2 28]]
Iteration 4 ______________________________________________________________________
Label Spreading model: 60 labeled & 270 unlabeled (330 total)
precision recall f1-score support
0 1.00 1.00 1.00 22
1 0.96 1.00 0.98 22
2 1.00 0.96 0.98 27
3 0.96 1.00 0.98 25
4 0.86 1.00 0.93 19
5 0.96 0.87 0.92 31
6 1.00 0.97 0.99 35
7 1.00 1.00 1.00 31
8 0.92 0.96 0.94 25
9 0.88 0.85 0.86 33
accuracy 0.96 270
macro avg 0.95 0.96 0.96 270
weighted avg 0.96 0.96 0.96 270
Confusion matrix
[[22 0 0 0 0 0 0 0 0 0]
[ 0 22 0 0 0 0 0 0 0 0]
[ 0 0 26 1 0 0 0 0 0 0]
[ 0 0 0 25 0 0 0 0 0 0]
[ 0 0 0 0 19 0 0 0 0 0]
[ 0 0 0 0 0 27 0 0 0 4]
[ 0 1 0 0 0 0 34 0 0 0]
[ 0 0 0 0 0 0 0 31 0 0]
[ 0 0 0 0 1 0 0 0 24 0]
[ 0 0 0 0 2 1 0 0 2 28]]
# Authors: The scikit-learn developers
# SPDX-License-Identifier: BSD-3-Clause
import matplotlib.pyplot as plt
import numpy as np
from scipy import stats
from sklearn import datasets
from sklearn.metrics import classification_report, confusion_matrix
from sklearn.semi_supervised import LabelSpreading
digits = datasets.load_digits()
rng = np.random.RandomState(0)
indices = np.arange(len(digits.data))
rng.shuffle(indices)
X = digits.data[indices[:330]]
y = digits.target[indices[:330]]
images = digits.images[indices[:330]]
n_total_samples = len(y)
n_labeled_points = 40
max_iterations = 5
unlabeled_indices = np.arange(n_total_samples)[n_labeled_points:]
f = plt.figure()
for i in range(max_iterations):
if len(unlabeled_indices) == 0:
print("No unlabeled items left to label.")
break
y_train = np.copy(y)
y_train[unlabeled_indices] = -1
lp_model = LabelSpreading(gamma=0.25, max_iter=20)
lp_model.fit(X, y_train)
predicted_labels = lp_model.transduction_[unlabeled_indices]
true_labels = y[unlabeled_indices]
cm = confusion_matrix(true_labels, predicted_labels, labels=lp_model.classes_)
print("Iteration %i %s" % (i, 70 * "_"))
print(
"Label Spreading model: %d labeled & %d unlabeled (%d total)"
% (n_labeled_points, n_total_samples - n_labeled_points, n_total_samples)
)
print(classification_report(true_labels, predicted_labels))
print("Confusion matrix")
print(cm)
# compute the entropies of transduced label distributions
pred_entropies = stats.distributions.entropy(lp_model.label_distributions_.T)
# select up to 5 digit examples that the classifier is most uncertain about
uncertainty_index = np.argsort(pred_entropies)[::-1]
uncertainty_index = uncertainty_index[
np.isin(uncertainty_index, unlabeled_indices)
][:5]
# keep track of indices that we get labels for
delete_indices = np.array([], dtype=int)
# for more than 5 iterations, visualize the gain only on the first 5
if i < 5:
f.text(
0.05,
(1 - (i + 1) * 0.183),
"model %d\n\nfit with\n%d labels" % ((i + 1), i * 5 + 10),
size=10,
)
for index, image_index in enumerate(uncertainty_index):
image = images[image_index]
# for more than 5 iterations, visualize the gain only on the first 5
if i < 5:
sub = f.add_subplot(5, 5, index + 1 + (5 * i))
sub.imshow(image, cmap=plt.cm.gray_r, interpolation="none")
sub.set_title(
"predict: %i\ntrue: %i"
% (lp_model.transduction_[image_index], y[image_index]),
size=10,
)
sub.axis("off")
# labeling 5 points, remote from labeled set
(delete_index,) = np.where(unlabeled_indices == image_index)
delete_indices = np.concatenate((delete_indices, delete_index))
unlabeled_indices = np.delete(unlabeled_indices, delete_indices)
n_labeled_points += len(uncertainty_index)
f.suptitle(
(
"Active learning with Label Propagation.\nRows show 5 most "
"uncertain labels to learn with the next model."
),
y=1.15,
)
plt.subplots_adjust(left=0.2, bottom=0.03, right=0.9, top=0.9, wspace=0.2, hspace=0.85)
plt.show()
Total running time of the script: (0 minutes 0.533 seconds)
Related examples
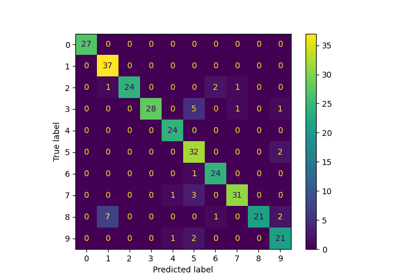
Label Propagation digits: Demonstrating performance